Machine Learning for Phase Transitions
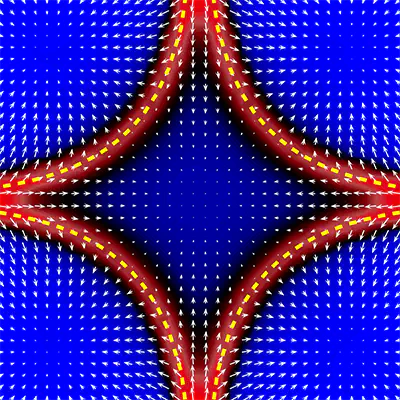
Artificial neural networks have successfully been used to identify phase transitions from data and classify data into distinct phases in an automated fashion. The power and success of these approaches (e.g., “learning by confusion” or the “prediction-based method”) can be attributed to the ability of deep neural networks to learn arbitrary functions. However, the larger a neural network, the more computational resources are needed to train it, and the more difficult it is to understand its decision making. In this project, we establish a solid theoretical foundation of popular machine-learning methods that rely on neural networks for detecting phase transitions and develop new efficient numerical routines to identify phase transitions directly from data. In the future, our methods could make it possible to detect as yet unknown phase transitions, for instance in quantum simulators or in novel materials.